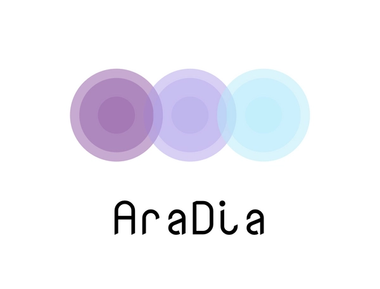
Data scarcity hinders Automatic Speech Recognition (ASR) performance on low-resource languages, especially when it comes to dysarthric speech. Challenges in collecting data from dysarthric speakers amplify the data scarcity issues with low-resource languages. In our project, we aim to tackle this problem by modifying the tempo and speed on healthy speech to generate dysarthric speech. This is further augmented using a cross-lingual Parallel Wave Generative (PWG) adversarial model trained on an English dysarthric dataset. We also propose a fine-tuning strategy for Arabic Conformer utilizing synthetically generated dysarthric speech at different severity levels. Furthermore, we utilise the Conformer’s character-level confusion to build a text correction module for Arabic dysarthric speech.